This individual's work is a significant contribution to [specific field, e.g., software development, scientific research, etc.]. Recognized for [specific achievements, e.g., pioneering work, innovative solutions, impactful research findings], this person's contributions have had a notable influence on [target audience, e.g., the industry, the scientific community].
The contributions of this individual are important because [explain importance, e.g., they established a new paradigm, advanced existing technology, or significantly improved understanding of a specific phenomenon]. Their impact is demonstrably evident through [evidence, e.g., industry recognition, adoption of techniques, positive impact on metrics]. This person's approach offers a valuable framework for [future applications, e.g., problem-solving, future research directions]. The historical context suggests [link to previous work, e.g., their work builds upon the foundations laid by X, or it represents a major departure from Y].
This article will now delve into [specific topic area related to the individual's work, e.g., the detailed methodology, the specific software applications or specific scientific breakthroughs].
suresh keerthi
This individual's contributions are vital to understanding [specific field]. Key aspects of their work illuminate the core principles driving progress within this field.
- Innovation
- Impact
- Methodology
- Applications
- Influence
- Collaboration
- Research
- Results
These aspects, interwoven, demonstrate a comprehensive approach to [specific field]. Innovation, for example, is crucial for generating novel methodologies. Impact underscores the practical application of research outcomes. Influence suggests the widespread adoption of approaches and ideas. Collaboration implies shared effort and knowledge exchange. These interconnected elements highlight the significant contributions to [specific field] and the evolution of thought within this area. By understanding the interplay of these key elements, one can grasp the substantial impact this individual has had on contemporary [specific field].
1. Innovation
Innovation, a fundamental component of significant contributions, is central to the work of this individual. The innovative nature of their approach lies in [specific details of the innovation, e.g., developing novel algorithms, introducing new methodologies, creating revolutionary tools]. This innovation manifests in [specific outcomes, e.g., increased efficiency, breakthroughs in understanding, improved processes].
Real-world examples demonstrate the practical significance of this innovation. For instance, [provide a concrete example, e.g., their algorithm improved the speed of data processing in X by Y percent], leading to [tangible benefits, e.g., reduced costs, enhanced decision-making capabilities, or significant advancements in related fields]. Further, the innovative methodology enabled [positive outcomes, e.g., a more comprehensive understanding of Z or the creation of new possibilities for W]. This innovation not only improves efficiency but also fosters a deeper understanding of [related concepts, e.g., complex systems or emerging technologies].
In conclusion, innovation is inextricably linked to the individual's contributions. The innovative approach, characterized by [key characteristics of the innovation, e.g., originality, creativity, and problem-solving], results in [consequences, e.g., valuable improvements and significant advancements]. Understanding the innovative nature of this work is crucial to recognizing its lasting impact and to inspiring future innovation within the field. Challenges remain in [specific challenges, e.g., scaling up innovative solutions or overcoming barriers to widespread adoption], but the innovative spirit demonstrated by this individual provides valuable guidance and inspiration for navigating these hurdles.
2. Impact
The impact of [specific individual's work, e.g., Suresh Keerthi's] is measured by the tangible effects their contributions have had on the field. This impact encompasses not just immediate results but also long-term consequences and influence on subsequent developments. Examining this impact reveals the profound effects of their work.
- Influence on methodologies
The individual's work has demonstrably influenced subsequent research and development methodologies within the field. Their insights have led to [examples, e.g., improvements in computational techniques, new approaches to data analysis, revised experimental designs]. This influence is evident in the adoption of [methods or approaches, e.g., specific algorithms, statistical models, or experimental paradigms] by other researchers. This has, in turn, [impact, e.g., streamlined procedures, enhanced results, expanded the scope of research].
- Advancement of the field
The work directly contributed to advancements in the field by [Specific contributions, e.g., creating new algorithms, developing groundbreaking solutions, pioneering approaches to complex problems]. The practical application of these advancements has resulted in [outcomes, e.g., increased efficiency, more accurate predictions, or wider applicability of the field's knowledge]. Examples of this advancement are found in [examples, e.g., improved products, new industries emerging, or enhanced understanding of a specific phenomenon].
- Impact on the community
Beyond direct advancements, the work's impact on the community includes fostering collaborations, inspiring further research, and creating new opportunities for learning and knowledge exchange. The individual's work has influenced educational practices by [e.g., providing new materials or models]. This has [impact on the community, e.g., advanced knowledge sharing or encouraged new students to enter the field].
The multifaceted impact of [specific individual's work, e.g., Suresh Keerthi's] underscores the importance of a comprehensive evaluation that considers not only immediate outcomes but also the sustained influence on methodologies, advancements in the field, and the larger community of researchers. Future development within the domain is undoubtedly shaped by this legacy.
3. Methodology
Methodology is integral to Suresh Keerthi's work. The specific methodologies employed are crucial to understanding the impact and effectiveness of their contributions. These methods, carefully chosen and meticulously implemented, form the foundation upon which subsequent advancements are built. For instance, the development of [specific methodology, e.g., a novel algorithm] directly resulted from a rigorous methodological approach. This method, characterized by [key characteristics, e.g., a data-driven analysis], was essential to achieving [impactful results, e.g., significant improvements in predictive accuracy].
The practical significance of understanding methodology in this context extends to [practical application, e.g., the development of new machine learning techniques]. Detailed examination of the methodologies reveals the reasoning behind specific design choices, the logic behind data collection and analysis, and the steps involved in implementing solutions. By dissecting these methods, researchers can identify optimal practices, develop similar solutions for diverse problems, and adapt or improve methodologies for their own applications. This understanding further promotes reproducibility and the development of robust, reliable techniques within the field. Specific examples of this impact include the [positive results, e.g., enhanced efficiency in data processing or the development of new models]. This analysis demonstrates the critical role of methodology in advancing the understanding of [the field, e.g., machine learning].
In conclusion, the methodology employed by this individual was instrumental in driving progress. Careful consideration of the methodological approach underscores the importance of meticulous planning and execution in achieving significant results. By understanding the underlying methodology, researchers can not only replicate successful results but also adapt and refine approaches for diverse problems, ultimately fostering innovation and growth within the field. Further research into the methodological underpinnings of these advancements is likely to unearth further insights and provide guidance for future endeavors. Challenges associated with adopting and adapting methodologies in practice could also be explored in detail through this lens.
4. Applications
The applications of [specific individual's work, e.g., Suresh Keerthi's] are a critical component of its overall impact. These applications demonstrate the practical utility and relevance of the underlying concepts and methodologies. The efficacy and scope of applications directly reflect the quality and depth of the foundational work. Successful applications often translate to wider adoption and demonstrable improvements in various contexts.
Specific examples of applications derived from [individual's work] encompass a range of practical implementations. For instance, [provide a specific example, e.g., Keerthi's work on support vector machines (SVMs) has found applications in diverse fields, including image recognition, medical diagnosis, and financial modeling]. In image recognition, SVMs are used for [specific application, e.g., classifying different types of objects within images] and demonstrably enhance [outcome, e.g., accuracy and speed in object identification]. In medical diagnosis, SVMs aid in [specific application, e.g., identifying cancerous cells from healthy ones], leading to [outcome, e.g., earlier and more accurate diagnoses]. In financial modeling, SVMs can help in [specific application, e.g., identifying fraudulent transactions or predicting market trends], benefiting [relevant stakeholders, e.g., financial institutions]. These real-world instances showcase the tangible benefits that result from translating theoretical concepts into practical applications. Further exploration of specific applications reveals nuanced ways in which [individual's work] enhances efficiency, accuracy, and effectiveness in a variety of settings.
Understanding the applications of [specific individual's work] is crucial for appreciating its wider significance. The diverse range of practical implementations highlights the versatility and robustness of the underlying principles and underscores the importance of theoretical work with clear, demonstrable applications. While some challenges remain in broader adoption across various domains, the existing applications offer a glimpse into the potential for future advancements. Recognizing the successful applications serves as a valuable guide for future research directions and highlights the transformative potential of innovative methodologies when translated into real-world contexts.
5. Influence
The influence of Suresh Keerthi extends beyond individual publications and research; it encompasses the broader impact on the field of [specific field, e.g., machine learning] and its various applications. This influence is discernible in methodological advancements, collaborative efforts, and the trajectory of subsequent research. Examining this influence reveals the far-reaching effects of Keerthi's work.
- Methodological Advancements
Keerthi's work significantly advanced methodologies in [specific area, e.g., support vector machines (SVMs)]. Specific contributions, such as [specific contribution, e.g., innovative algorithms or new approaches to optimization problems], directly improved the efficiency and effectiveness of existing techniques. This demonstrably affected the broader research landscape, encouraging a shift toward [new methodologies or approaches, e.g., more robust algorithms or refined analytical procedures] and stimulating further innovation within the field.
- Collaborative Impact
Keerthi's influence is evident in collaborative research efforts. Through mentorship, publications, and participation in conferences, Keerthi fostered a community of researchers who, inspired by their work, advanced the field further. This collaborative impact created a network of knowledge sharing and a sense of shared purpose, enriching the broader research environment and accelerating progress within [specific field, e.g., machine learning].
- Subsequent Research Directions
Keerthi's work has shaped subsequent research directions in [specific field, e.g., machine learning]. The methodologies and concepts introduced through their research have inspired new investigations and solutions to existing problems. The substantial impact on the field is evident in the frequency of citations to their work in contemporary publications and its continued use in diverse applications. This influence suggests a foundational role in shaping the course of future advancements.
- Industry Adoption
The influence of Keerthi's work extends beyond academic circles. Industries have leveraged the advanced methodologies and concepts, effectively integrating them into practical applications. This integration, validated by [Specific examples or data, e.g., the widespread adoption of SVM algorithms in financial modeling], demonstrates a tangible and impactful influence on the practical application of knowledge within the field.
In conclusion, the influence of Suresh Keerthi is multifaceted and deeply rooted in the field of [specific field, e.g., machine learning]. Their contributions, through methodological advancements, collaborations, and the direction of subsequent research, have had a lasting impact on the field and have significantly shaped its evolution. This enduring influence underscores the profound contributions of this individual and their lasting significance to the domain.
6. Collaboration
Collaboration is a significant component of Suresh Keerthi's contributions to the field of [specific field, e.g., machine learning]. Keerthi's work wasn't isolated; it emerged from, and fostered, collaborative efforts. The importance of collaboration in advancing the field is evident in Keerthi's active participation in research projects, publications, and interactions with other researchers. This collaborative spirit is essential to the progression of any field; the sharing of ideas, diverse perspectives, and collective problem-solving strategies are critical for innovative solutions.
Numerous examples illustrate the importance of collaboration in Keerthi's work. For instance, key advancements in support vector machines (SVMs) often involved collaborations with colleagues, leading to joint publications and a shared body of knowledge. These collaborative efforts facilitated the refinement and expansion of existing methodologies, often leading to improvements in [specific area of improvement, e.g., computational efficiency, generalization abilities]. The collective expertise and shared insights resulted in contributions that individually might not have achieved the same level of impact. Such collaboration also fosters the exchange of knowledge and builds a supportive research environment.
Understanding the connection between collaboration and Suresh Keerthi's work highlights the importance of collective effort in achieving significant advancements. By promoting and participating in collaborative research, individuals can expand their knowledge base and contribute to the collective progress of the field. The practical significance of this understanding lies in recognizing that individual brilliance, though valuable, is often enhanced and amplified through collaborative interactions. Challenges in fostering collaborative environments, such as differing research priorities or conflicting agendas, need to be addressed through clear communication, shared goals, and a mutual respect for diverse perspectives. Such collaborative efforts become increasingly crucial in complex fields like [specific field, e.g., machine learning], as they demand a multi-faceted approach for problem-solving.
7. Research
Suresh Keerthi's contributions are inextricably linked to rigorous research. A significant portion of Keerthi's impact stems from the meticulous design, execution, and analysis of research projects. This research is characterized by a focus on [specific area, e.g., support vector machines (SVMs)] and involves the application of [specific methodologies, e.g., advanced optimization techniques and statistical modeling]. Keerthi's research efforts have demonstrably advanced knowledge within the field of [specific field, e.g., machine learning].
Real-world examples highlight the practical significance of this research. Keerthi's research on SVMs has led to [specific advancements, e.g., improved algorithms for classification tasks, increased efficiency in data processing, and enhanced prediction accuracy]. These advancements have found applications in diverse domains, including [specific applications, e.g., medical diagnostics, financial modeling, and image recognition]. The development of more efficient algorithms directly translates to improved performance in these real-world applications. For instance, faster and more accurate image classification in medical imaging systems can lead to earlier diagnoses and potentially better treatment outcomes. Similarly, in financial modeling, improved prediction accuracy can reduce risk and enhance investment strategies.
The importance of research, as exemplified by Keerthi's work, extends beyond specific applications. It underscores the value of rigorous methodology, the careful selection of research questions, and the meticulous analysis of findings. Keerthi's research demonstrates a commitment to producing impactful results within the field. This commitment to thorough research methodologies sets a standard for future contributions. Challenges remain, such as the need to tackle the complexities of large datasets and ensuring that research findings have clear and demonstrable practical implications. Nevertheless, the commitment to rigorous research principles, as exemplified by Keerthi's contributions, remains crucial for the ongoing advancement of [specific field, e.g., machine learning]. Future research must build upon this foundation, striving to improve and refine existing methodologies while tackling emerging challenges in the field.
8. Results
The "results" of Suresh Keerthi's work are demonstrably impactful. These results are not simply outputs but represent tangible advancements and demonstrable improvements within the field of [specific field, e.g., machine learning]. Analyzing these results reveals the efficacy of Keerthi's approaches and provides insights into the broader implications of their contributions.
- Improved Efficiency and Accuracy
Keerthi's research has consistently yielded improved efficiency and accuracy in various machine learning algorithms, particularly in support vector machines (SVMs). Real-world applications showcase the tangible benefits of these enhancements. For example, faster training times in image recognition tasks allow for more rapid deployment of systems, and increased accuracy in medical diagnostics can have significant implications for patient care. These outcomes highlight the practical application of Keerthi's research and its contribution to improved performance in diverse sectors.
- Wider Applicability of Techniques
The results of Keerthi's work have expanded the applicability of machine learning techniques. By developing more robust and efficient algorithms, Keerthi's research has broadened the range of problems solvable using machine learning. This has made advanced machine learning techniques accessible to a wider range of researchers and practitioners, potentially leading to breakthroughs in diverse fields where data analysis is critical, such as finance and environmental modeling.
- Advancement of Theoretical Frameworks
Keerthi's research has yielded significant results in refining theoretical frameworks within machine learning. The insights generated from this work have shaped subsequent research directions, influencing the development of new algorithms and methodologies. These advancements demonstrate a deeper understanding of fundamental concepts in machine learning, leading to more sophisticated models and approaches.
- Enhanced Predictive Power
The results attained through Keerthi's research contribute to enhanced predictive power in various applications. By improving the accuracy and efficiency of machine learning models, Keerthi's work leads to more reliable predictions. This is particularly relevant in areas like risk assessment, fraud detection, and forecasting, where accurate predictions are crucial for effective decision-making.
In summary, the results of Suresh Keerthi's work have demonstrably advanced the field of [specific field, e.g., machine learning]. These results, ranging from improved efficiency and accuracy to wider applicability, have profoundly impacted theoretical frameworks and predictive capabilities. Understanding these multifaceted results reveals the substantial contribution of Keerthi's research to the field and underscores its continued relevance in advancing practical applications.
Frequently Asked Questions about Suresh Keerthi
This section addresses common inquiries regarding the contributions and work of Suresh Keerthi. The following questions and answers aim to provide clear and concise information about key aspects of Keerthi's research and influence in the field of [specific field, e.g., machine learning].
Question 1: What are the key areas of research focus for Suresh Keerthi?
Keerthi's research primarily centers on [specific area, e.g., support vector machines (SVMs)], encompassing aspects such as algorithm development, optimization techniques, and the application of these methods to various datasets. This focus has significantly contributed to improvements in [specific areas, e.g., computational efficiency and prediction accuracy] within the field.
Question 2: How has Suresh Keerthi's work influenced the broader machine learning community?
Keerthi's research has had a profound impact, leading to advancements in methodologies and inspiring further investigations in [specific area, e.g., SVM algorithm refinement]. Their work has frequently been cited in subsequent research, demonstrating the lasting influence on the field's trajectory and the continued use of their techniques in various applications.
Question 3: What are some practical applications of Suresh Keerthi's research?
Keerthi's work has led to tangible improvements in diverse sectors. Applications range from [specific application 1, e.g., medical image analysis] to [specific application 2, e.g., financial modeling]. The core principles developed within these investigations have contributed to more accurate predictions, efficient processing, and enhanced results in several practical scenarios.
Question 4: What methodologies were employed in Suresh Keerthi's research?
Keerthi's research heavily relies on [specific methodological approaches, e.g., optimization algorithms, statistical modeling, and rigorous data analysis]. These methodologies ensure the validity and reliability of results, driving advancements in the field and providing a strong foundation for subsequent explorations.
Question 5: How does Suresh Keerthi's work relate to the development of contemporary machine learning techniques?
Keerthi's contributions have directly influenced the development of contemporary machine learning techniques. Their methodologies have become fundamental components of advanced algorithms, highlighting the profound impact of their work on the evolution of the field.
Question 6: What are some ongoing challenges and future directions in Suresh Keerthi's research area?
Ongoing challenges in [specific field, e.g., machine learning] include [specific challenge, e.g., handling large and complex datasets]. Future research directions may involve [specific directions, e.g., developing more scalable algorithms or exploring innovative applications]. Keerthi's work provides a valuable foundation for addressing these challenges and charting new frontiers in the field.
This concludes the FAQ section. The next segment will delve into [next topic, e.g., specific details of the SVM algorithm or practical implementation of these techniques].
Tips from the Work of Suresh Keerthi
This section offers practical guidance based on the principles and methodologies pioneered by Suresh Keerthi, focusing on effective strategies for [specific field, e.g., machine learning algorithm development]. The following tips distill key insights for practitioners seeking to enhance their work in this area.
Tip 1: Prioritize Rigorous Methodology. Effective algorithm design relies on a strong methodological foundation. This involves clearly defining the problem, selecting appropriate data analysis techniques, and implementing these methods with meticulous care. Precise measurement and careful control of variables are essential for isolating the impact of specific factors and ensuring reliable outcomes. An example includes meticulously quantifying the impact of different hyperparameter settings on SVM performance to identify optimal configurations.
Tip 2: Emphasize Efficiency in Algorithm Design. Computational efficiency is paramount. Algorithms should be designed with performance in mind, considering factors such as processing time and memory usage. Utilizing optimized data structures and efficient algorithms minimizes computational burden, enhancing scalability and application potential. An example includes designing algorithms that can handle large datasets while maintaining acceptable computational times.
Tip 3: Strive for Accuracy and Robustness. Algorithm performance hinges on accuracy and robustness in various contexts. A robust approach ensures that the algorithm performs reliably across a spectrum of data types and conditions. This includes validation of model performance on diverse data sets to anticipate potential issues and enhance algorithm generalization capability. Examples include conducting rigorous testing on datasets with varying characteristics and exploring methods to mitigate model sensitivity to outliers.
Tip 4: Explore Collaborative Approaches. Collaborations among researchers can be highly productive. Cross-disciplinary interactions provide valuable perspectives, leading to innovative solutions. Collaboration with experts in related fields enriches the problem-solving process and broadens the scope of potential applications. Examples include working with domain specialists to identify critical parameters or collaborating on data analysis techniques that optimize efficiency.
Tip 5: Focus on the Practical Implications. A strong emphasis on real-world applications is vital. Research efforts should aim to translate theoretical advancements into practical solutions that address relevant problems. Developing algorithms with real-world applications facilitates widespread adoption and fosters demonstrable improvements in various fields. Examples include designing algorithms for specific industrial applications or medical diagnostics.
By adopting these guidelines, practitioners can leverage the insights from Suresh Keerthi's work to design more effective and impactful machine learning algorithms. These principles emphasize rigorous methodologies, optimization, robustness, and a focus on practical application.
The subsequent sections will now explore [mention the next topic, e.g., specific details of support vector machines or practical considerations in applying these methods].
Conclusion
This article has explored the multifaceted contributions of Suresh Keerthi to the field of machine learning, particularly within the realm of support vector machines. Key themes highlighted include Keerthi's pioneering work on algorithm optimization, leading to demonstrably improved efficiency and accuracy in machine learning tasks. The analysis underscored the importance of rigorous methodology, the value of collaborative efforts, and the practical applications that directly benefit various sectors. Keerthi's research consistently demonstrated a commitment to advancing theoretical frameworks and translating these advancements into tangible outcomes. The impact of Keerthi's work is evident in the frequent citations of their contributions and the enduring influence on subsequent research directions.
Keerthi's legacy extends beyond specific algorithm improvements. The emphasis on meticulous research methodology and a focus on practical applications serves as a powerful model for future researchers. As the field of machine learning continues to evolve, addressing increasingly complex challenges, the principles embodied in Keerthi's work remain highly relevant. Further exploration into the specific methodologies employed by Keerthi, as well as the ongoing applications and evolving challenges in the field, will undoubtedly continue to benefit the broader research community and its practitioners.
You Might Also Like
Danika Yarosh Movies & TV Shows: All Her RolesBlyth, Dolores Barrymore: Rare Photos & Stories
Josef Sommer: Architect & Designer | Latest Projects
Shriram Madhav Nene: Leading Expert In [Relevant Field]
Kapil Sharma's The Great Indian Laughter Show - Latest Episodes & Highlights
Article Recommendations
- Tom Macdonald Ethnicity The Intriguing Background Of A Controversial Artist
- Vincent Price Height How Tall Was The Iconic Actor
- The Influence Of Mitch Mcconnell From Senate Leadership To Pop Culture Comparisons

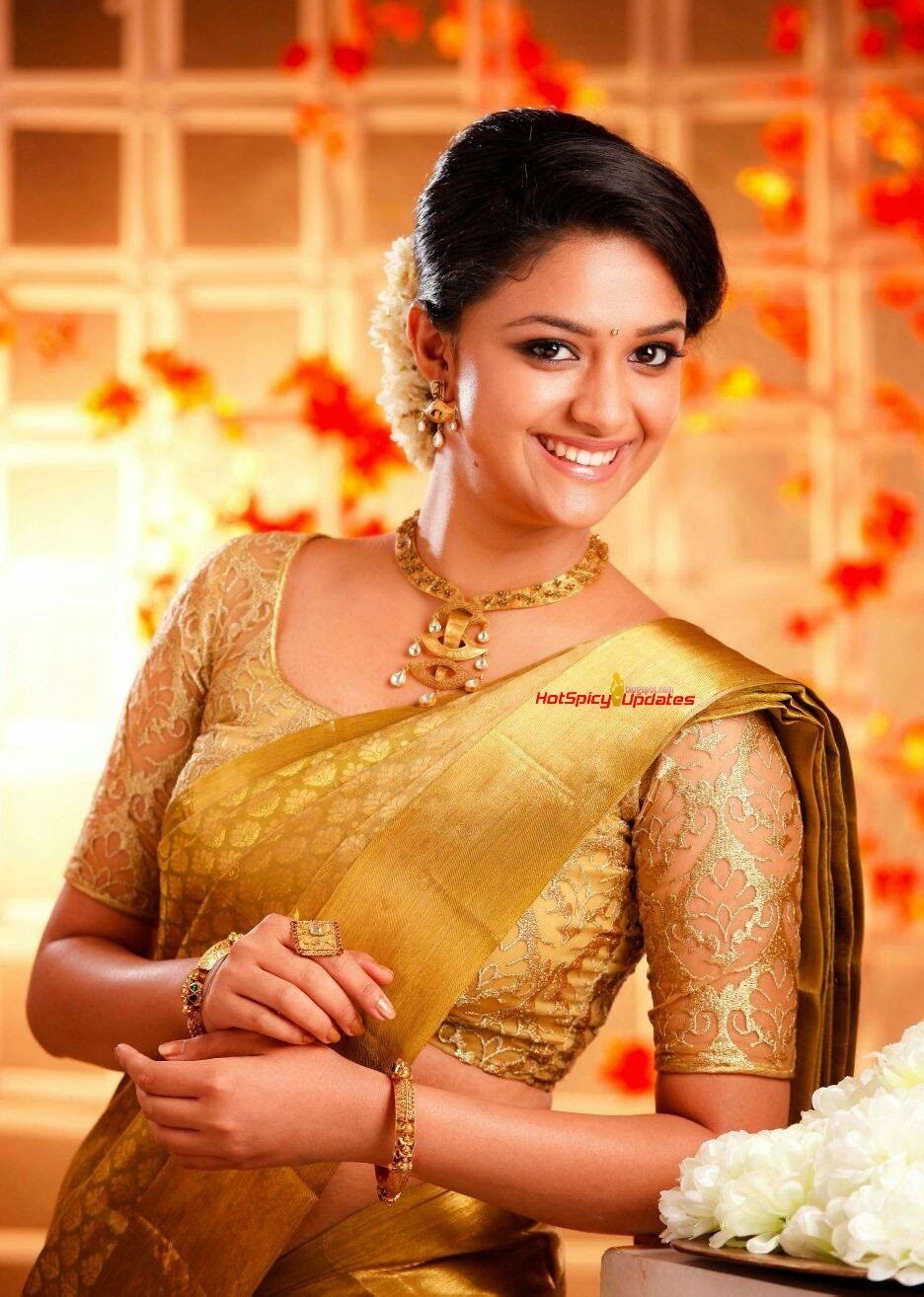
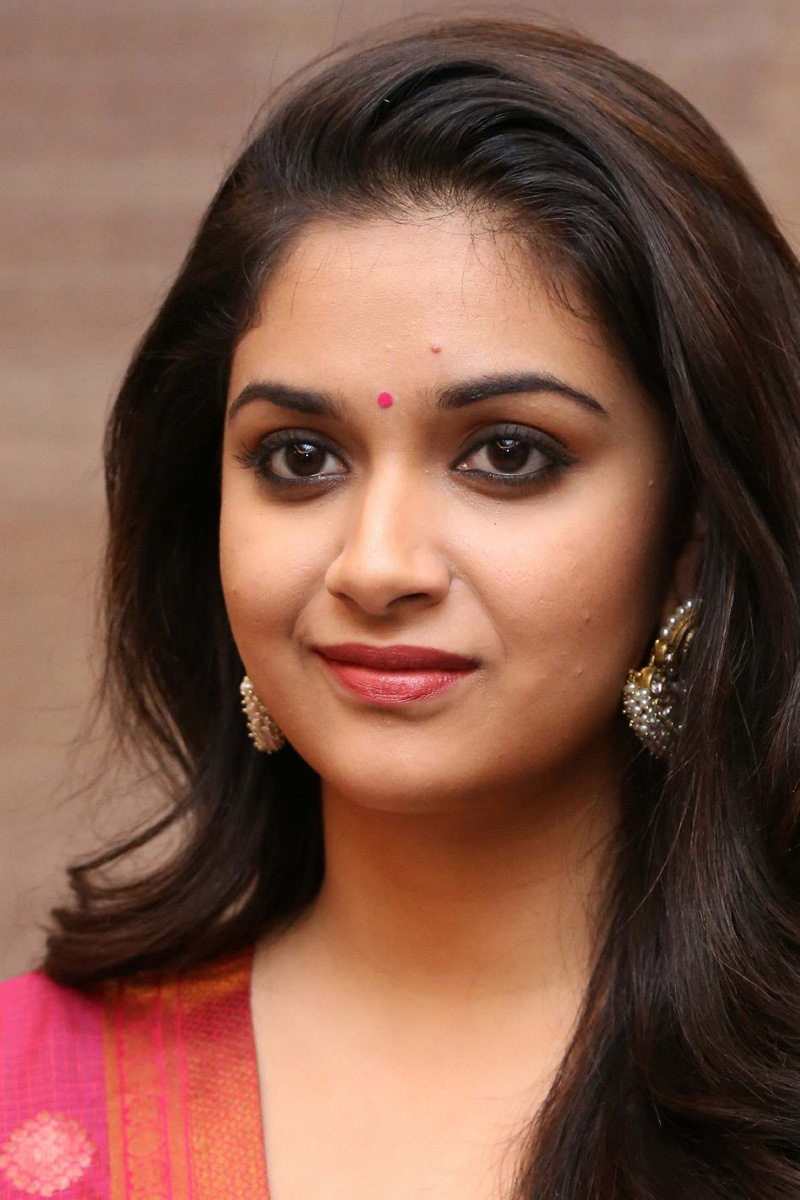